6 Critical AI Ethics Challenges: Navigating the Future of Artificial Intelligence
The Dawn of Ethical AI: Charting a Responsible Course
As we stand on the brink of an AI-driven future, the ethical implications of artificial intelligence loom large. The rapid advancement of AI technologies has ushered in an era of unprecedented possibilities, but with great power comes great responsibility. The AI ethics challenges we face today are not merely academic exercises; they are urgent, real-world issues that will shape the future of humanity.
From the boardrooms of tech giants to the halls of academia, the conversation around AI ethics challenges is gaining momentum. These challenges touch every aspect of our lives – from the algorithms that influence our daily decisions to the autonomous systems that may one day make life-or-death choices. As we navigate this complex landscape, we must ask ourselves: How can we harness the power of AI while ensuring it aligns with our values and ethical principles?
In this comprehensive exploration, we’ll delve into six critical AI ethics challenges that demand our attention. Each challenge represents a crucial battleground where the future of AI will be decided. Whether you’re a tech enthusiast, a policy maker, or simply a concerned citizen, understanding these challenges is key to shaping an AI future we can all embrace.
Ready to embark on this journey through the ethical maze of AI? Let’s begin by exploring the key areas we’ll cover. Take a moment to review the table of contents below – it’s your roadmap to understanding the complex world of AI ethics challenges.
- Bias and Fairness in AI Systems
- The Algorithmic Prejudice: Unmasking Hidden Biases
- Equality by Design: Building Fairness into AI
- Diverse Data, Diverse Outcomes: The Key to Equitable AI
- Transparency and Explainability
- Cracking the Black Box: The Quest for Interpretable AI
- From Complexity to Clarity: Making AI Decisions Understandable
- The Right to Explanation: Empowering Users in the Age of AI
- Privacy and Data Protection
- Data Guardians: Safeguarding Personal Information in AI Systems
- The Consent Conundrum: Navigating Privacy in the AI Era
- Anonymity in the Algorithm: Preserving Privacy Without Sacrificing Utility
- Accountability and Liability
- Who’s at the Wheel?: Determining Responsibility in AI Decision-Making
- Ethics by Design: Embedding Accountability into AI Systems
- AI Security and Misuse Prevention
- Digital Fortresses: Protecting AI Systems from Malicious Attacks
- The Double-Edged Sword: Preventing AI Weaponization
- Ethical Decision-Making in Autonomous Systems
- Silicon Morality: Teaching Ethics to Machines
- The Trolley Problem 2.0: Ethical Dilemmas in AI-Driven Vehicles
- Beyond Asimov: Developing Comprehensive Ethical Frameworks for AI
- Conclusion: Addressing AI Ethics Challenges
Now, let’s dive into the first major challenge in our exploration of AI ethics.
Bias and Fairness in AI Systems
As we delve into the world of AI ethics challenges, perhaps no issue is more pressing or pervasive than the question of bias and fairness. AI systems, despite their promise of objectivity, can often perpetuate and even amplify existing societal biases. This section explores the multifaceted nature of this challenge and the efforts to create more equitable AI.
The Algorithmic Prejudice: Unmasking Hidden Biases
At the heart of many AI ethics challenges lies the insidious problem of algorithmic bias. These biases, often unintentional but deeply impactful, can creep into AI systems through various means – from skewed training data to flawed algorithm design. The result? AI systems that make unfair or discriminatory decisions, affecting everything from hiring practices to criminal justice outcomes.
Addressing this AI ethics challenge requires a keen eye for detecting bias and a commitment to fairness. Researchers and ethicists are developing sophisticated tools to audit AI systems for bias, examining not just the outcomes but the underlying data and algorithms. This process of „unmasking” hidden biases is crucial in building trust in AI systems and ensuring they serve all members of society equitably.
The challenge of algorithmic prejudice underscores a fundamental AI ethics challenge: how to create systems that are not just powerful, but also fair and just. As we continue to grapple with this issue, it’s clear that overcoming algorithmic bias will require ongoing vigilance, innovative technical solutions, and a deep commitment to ethical AI development.
Equality by Design: Building Fairness into AI
The concept of „Equality by Design” represents a proactive approach to AI ethics challenges. Rather than treating fairness as an afterthought, this philosophy advocates for embedding ethical considerations into the very fabric of AI systems from the ground up. It’s a shift from asking „How can we make this AI system fair?” to „How can we design an AI system that is inherently fair?”
This approach to AI ethics challenges requires a fundamental rethinking of how we develop AI. It involves creating new algorithms that balance multiple objectives simultaneously – not just accuracy and efficiency, but also fairness and equality. Developers are exploring techniques like adversarial debiasing and fairness constraints to create AI systems that are both powerful and equitable.
However, implementing Equality by Design is not without its own AI ethics challenges. Defining „fairness” in a way that can be operationalized in code is a complex task, often involving difficult trade-offs. Moreover, ensuring that efforts to promote equality don’t inadvertently create new forms of bias requires constant vigilance and testing. As we navigate these challenges, collaboration between technologists, ethicists, and affected communities will be crucial in creating AI systems that truly serve the interests of all.
Diverse Data, Diverse Outcomes: The Key to Equitable AI
One of the most critical AI ethics challenges in achieving fairness lies in the data used to train AI systems. The adage „garbage in, garbage out” takes on new significance when we consider the impact of biased or unrepresentative data on AI outcomes. Ensuring diversity in AI training data is not just a technical challenge, but a social and ethical imperative.
This AI ethics challenge goes beyond simply collecting more data from underrepresented groups. It involves understanding the historical and social contexts of data, recognizing potential biases in data collection methods, and developing strategies to compensate for gaps in datasets. Researchers are exploring innovative approaches like synthetic data generation and transfer learning to address these issues, each bringing its own set of ethical considerations.
Addressing the challenge of data diversity also highlights the importance of diversity in AI development teams. Diverse teams are more likely to recognize potential biases, consider a wider range of perspectives, and create more inclusive AI systems. As we work towards more equitable AI, fostering diversity and inclusion at every stage of the AI development process becomes not just an ethical imperative, but a practical necessity in overcoming AI ethics challenges.
Transparency and Explainability
In the realm of AI ethics challenges, the issues of transparency and explainability stand out as crucial concerns. As AI systems become more complex and influential, the need to understand their decision-making processes grows ever more pressing. This section explores the challenges and potential solutions in making AI more transparent and explainable.
Cracking the Black Box: The Quest for Interpretable AI
One of the most significant AI ethics challenges is the „black box” nature of many advanced AI systems, particularly deep learning models. These systems can produce highly accurate results, but their internal workings often remain opaque even to their creators. This lack of interpretability raises serious ethical concerns, especially when AI is used in high-stakes decision-making processes.
The quest for interpretable AI is at the forefront of addressing this AI ethics challenge. Researchers are developing techniques like LIME (Local Interpretable Model-agnostic Explanations) and SHAP (SHapley Additive exPlanations) to provide insights into how AI systems arrive at their decisions. These efforts aim to make AI more transparent without sacrificing performance, a delicate balance that lies at the heart of many AI ethics challenges.
As we work towards cracking the AI black box, we must also consider the ethical implications of interpretability itself. How much transparency is enough? How do we balance the need for explainability with the protection of proprietary algorithms? These questions underscore the complexity of AI ethics challenges in the pursuit of interpretable AI.
From Complexity to Clarity: Making AI Decisions Understandable
Another crucial aspect of AI ethics challenges is making AI decisions understandable to non-experts. As AI systems increasingly impact our daily lives, from content recommendations to credit decisions, there’s a growing ethical imperative to ensure that affected individuals can comprehend the reasoning behind these decisions. This AI ethics challenge involves not just technical solutions, but also effective communication strategies.
Researchers and designers are exploring various approaches, from visual explanations to natural language interfaces, to bridge the gap between complex AI processes and human understanding. The goal is to create AI systems that can explain their decisions in terms that are meaningful and actionable for users. However, simplifying complex AI decisions without losing crucial nuances presents its own set of AI ethics challenges, requiring a careful balance between accessibility and accuracy.
The Right to Explanation: Empowering Users in the Age of AI
The concept of a „right to explanation” has emerged as a key consideration in AI ethics challenges. This principle asserts that individuals have a right to understand how AI systems make decisions that affect them. It’s a response to the growing concern that AI systems are making increasingly important decisions about our lives without sufficient transparency or accountability.
Implementing the right to explanation presents several AI ethics challenges. How detailed should explanations be? How can we ensure explanations are accessible to diverse audiences? And how do we balance the need for transparency with the protection of trade secrets and intellectual property? As we grapple with these questions, it’s clear that the right to explanation will play a crucial role in shaping the ethical landscape of AI development and deployment.
Privacy and Data Protection
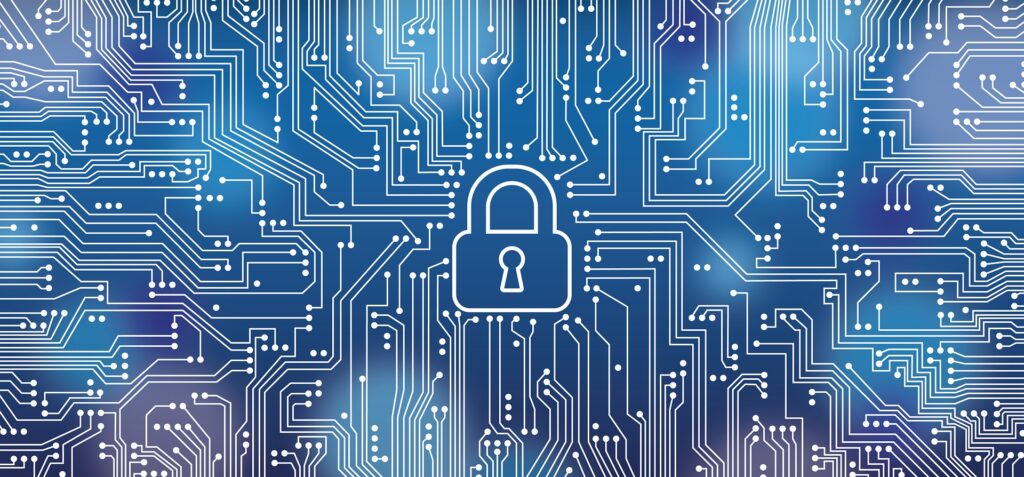
Data Guardians: Safeguarding Personal Information in AI Systems
The proliferation of AI systems has brought privacy and data protection to the forefront of AI ethics challenges. AI’s voracious appetite for data, combined with its ability to process and analyze vast amounts of information, raises significant concerns about the protection of personal information. This challenge is compounded by the fact that AI systems can often infer sensitive information from seemingly innocuous data.
Addressing this AI ethics challenge requires robust data protection measures and ethical guidelines for data usage. It involves developing AI systems that can learn from data while respecting individual privacy, a task that often involves complex trade-offs between utility and privacy. As we navigate these challenges, the role of „data guardians” – professionals dedicated to safeguarding personal information in AI systems – becomes increasingly crucial.
The Consent Conundrum: Navigating Privacy in the AI Era
One of the most pressing AI ethics challenges in the realm of privacy is the issue of informed consent. Traditional models of consent are often inadequate in the face of AI’s complex and often opaque data processing capabilities. Users may agree to share certain data without fully understanding how AI systems might use or interpret that information.
This AI ethics challenge is further complicated by the dynamic nature of AI systems. As these systems learn and evolve, their use of data may change over time, potentially exceeding the scope of the original consent. How can we ensure that consent remains meaningful and informed in such a fluid environment?
Addressing this conundrum requires innovative approaches to consent mechanisms. Some proposed solutions include dynamic consent models that allow users to update their preferences over time, or AI-powered tools that help users understand the implications of their data sharing decisions. As we grapple with these AI ethics challenges, the goal is to empower individuals with genuine control over their personal information in the AI age.
Anonymity in the Algorithm: Preserving Privacy Without Sacrificing Utility
The challenge of maintaining anonymity in AI systems presents a complex set of AI ethics challenges. On one hand, anonymization techniques are crucial for protecting individual privacy. On the other, excessive anonymization can reduce the utility of data for AI systems, potentially limiting their effectiveness and societal benefits.
This balancing act is further complicated by the power of modern AI systems to de-anonymize data through sophisticated analysis and cross-referencing. What was once considered sufficiently anonymized data may no longer be secure in the face of advanced AI techniques. This creates a moving target for privacy protection, requiring constant innovation in anonymization methods.
Researchers are exploring various approaches to address these AI ethics challenges, including differential privacy techniques and federated learning. These methods aim to preserve the utility of data for AI systems while providing strong privacy guarantees. However, implementing these techniques at scale and across diverse AI applications remains a significant challenge. As we continue to navigate this landscape, the ethical imperative is clear: we must strive to harness the power of AI while steadfastly protecting individual privacy.
Accountability and Liability
Who’s at the Wheel?: Determining Responsibility in AI Decision-Making
As AI systems become increasingly autonomous and influential in decision-making processes, one of the most pressing AI ethics challenges is determining who should be held accountable when things go wrong. This question of responsibility is not just a philosophical one, but has real-world implications for legal liability, ethical governance, and public trust in AI technologies.
The challenge lies in the distributed nature of AI development and deployment. Multiple parties are often involved – from data providers and algorithm designers to system integrators and end-users. When an AI system makes a harmful decision, it’s not always clear where the responsibility lies. This ambiguity in accountability presents significant AI ethics challenges, potentially leading to a situation where no one takes responsibility for AI-related harms.
Addressing this challenge requires developing clear frameworks for AI accountability. This might involve creating new legal and regulatory structures that can adequately address the unique challenges posed by AI systems. It also necessitates fostering a culture of responsibility in AI development, where all stakeholders are aware of their roles and potential liabilities. As we navigate these AI ethics challenges, the goal is to ensure that as AI systems become more powerful, they also become more accountable.
Interestingly, the push for greater AI accountability is also creating new job opportunities. As organizations grapple with these AI ethics challenges, new roles are emerging, such as AI ethicists, algorithmic auditors, and AI compliance officers. These professionals are tasked with ensuring that AI systems are developed and deployed responsibly, creating a new field at the intersection of technology, ethics, and law. This trend underscores how addressing AI ethics challenges not only safeguards against potential harms but also drives innovation and creates new avenues for professional development in the AI era.
Ethics by Design: Embedding Accountability into AI Systems
A proactive approach to AI ethics challenges involves embedding accountability mechanisms directly into AI systems from the ground up – a concept known as „Ethics by Design.” This approach aims to create AI systems that are not only powerful and efficient but also inherently accountable and ethically aligned.
Implementing Ethics by Design involves several key strategies. First, it requires developing AI systems with built-in audit trails, allowing for thorough examination of decision-making processes. Second, it involves creating AI systems with the ability to explain their decisions in human-understandable terms, addressing the „black box” problem. Third, it necessitates incorporating ethical constraints directly into AI algorithms, ensuring that AI systems operate within predefined ethical boundaries.
However, implementing Ethics by Design is not without its own AI ethics challenges. It requires careful consideration of what ethical principles should be embedded and how they should be prioritized. It also raises questions about who gets to define these ethical constraints and how they can be updated as societal values evolve. Despite these challenges, Ethics by Design represents a promising approach to creating more accountable and trustworthy AI systems, addressing many of the core AI ethics challenges we face today.
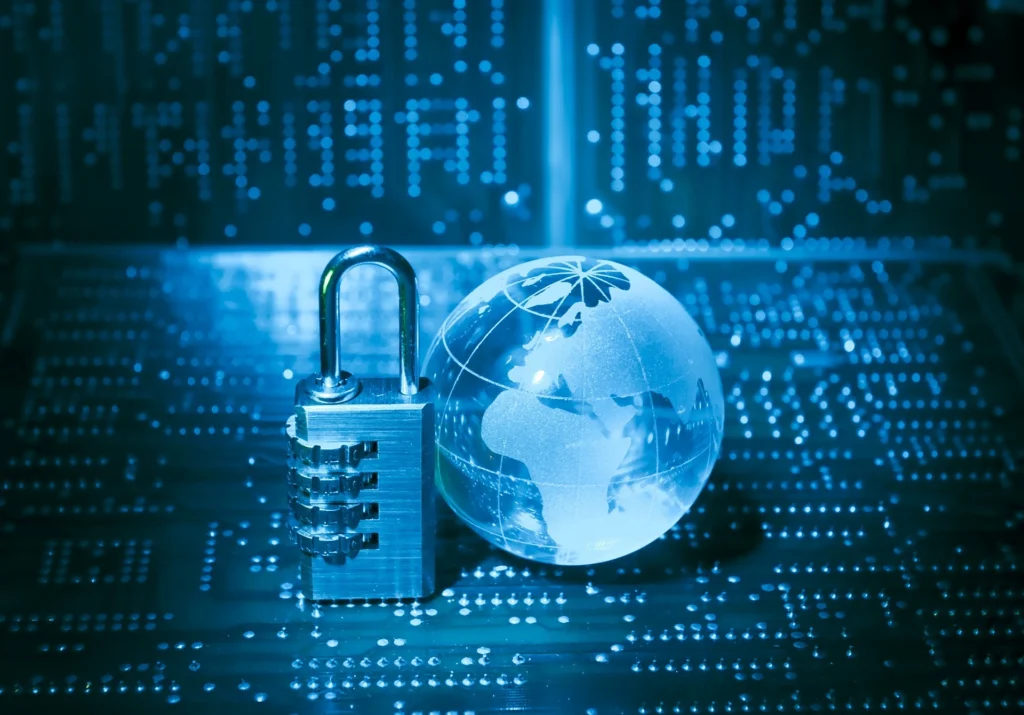
AI Security and Misuse Prevention
Digital Fortresses: Protecting AI Systems from Malicious Attacks
As AI systems become more prevalent and powerful, they also become attractive targets for cyberattacks, presenting a new frontier in AI ethics challenges. The security of AI systems is not just a technical issue but an ethical imperative, as compromised AI could lead to severe consequences ranging from privacy breaches to critical infrastructure failures.
One of the primary AI ethics challenges in this domain is the development of robust defense mechanisms against adversarial attacks. These attacks can manipulate AI systems into making incorrect decisions or revealing sensitive information. Researchers are working on techniques like adversarial training and defensive distillation to make AI models more resilient, but the cat-and-mouse game between attackers and defenders continues to evolve.
Another crucial aspect of AI security involves protecting the integrity of training data and models. As AI systems often deal with sensitive information, ensuring the confidentiality and integrity of this data becomes a significant ethical and security challenge. Techniques like federated learning and homomorphic encryption are being explored to address these AI ethics challenges, allowing AI systems to learn from data while keeping it secure and private.
The Double-Edged Sword: Preventing AI Weaponization
The potential weaponization of AI technology represents one of the most serious AI ethics challenges we face. As AI capabilities advance, there’s growing concern about its potential use in autonomous weapons systems, cyber warfare, or as a tool for mass manipulation and disinformation.
Preventing the misuse of AI for harmful purposes requires a multi-faceted approach. On a technical level, it involves developing AI systems with built-in safeguards and limitations. On a policy level, it calls for international cooperation and the establishment of clear guidelines and regulations governing the development and use of AI in sensitive domains.
The AI ethics challenges in this area are complex and often involve difficult trade-offs. How do we balance the potential benefits of AI in defense and security with the risks of its misuse? How can we promote beneficial AI research while preventing its weaponization? Addressing these questions requires ongoing dialogue between technologists, ethicists, policymakers, and the public to ensure that AI development aligns with our collective values and ethical principles.
Ethical Decision-Making in Autonomous Systems
Silicon Morality: Teaching Ethics to Machines
One of the most profound AI ethics challenges lies in imbuing autonomous systems with the ability to make ethical decisions. As AI systems become more advanced and are deployed in increasingly complex environments, they are often required to make decisions with significant ethical implications. This raises the question: how do we teach machines to make moral choices?
Addressing this AI ethics challenge involves translating human ethical principles into a form that machines can understand and apply. Researchers are exploring various approaches, from rule-based systems to more flexible machine learning models trained on ethical decision-making scenarios. However, this process is fraught with difficulties, as human ethics are often context-dependent and can vary across cultures and individuals. The challenge lies not just in coding ethics into AI, but in deciding whose ethics should be encoded and how to handle ethical dilemmas where there may be no clear „right” answer.
The Trolley Problem 2.0: Ethical Dilemmas in AI-Driven Vehicles
The advent of autonomous vehicles has brought classic ethical dilemmas like the trolley problem into sharp focus, presenting one of the most tangible AI ethics challenges of our time. In scenarios where harm is unavoidable, how should an AI-driven vehicle decide who to protect or endanger? These decisions, which humans make instinctively in split seconds, must be pre-programmed into autonomous systems.
This AI ethics challenge extends beyond just the technical implementation. It raises profound questions about the values we want to embed in our AI systems and how these align with societal norms and individual preferences. Should an autonomous vehicle prioritize its passengers over pedestrians? Should it take into account factors like age or number of potential victims? As we grapple with these questions, we must also consider the legal and social implications of codifying such ethical decisions into AI systems, potentially setting precedents that could shape the future of AI ethics.
Beyond Asimov: Developing Comprehensive Ethical Frameworks for AI
While Asimov’s Three Laws of Robotics provided an early framework for thinking about AI ethics, the complexity of modern AI systems demands more comprehensive and nuanced ethical guidelines. Developing these frameworks represents one of the most crucial AI ethics challenges we face today. It requires not only technical expertise but also input from philosophers, ethicists, policymakers, and the public at large.
These ethical frameworks must be flexible enough to apply across diverse AI applications while still providing clear guidance for specific scenarios. They need to address issues ranging from fairness and transparency to privacy and accountability. Moreover, they must be adaptable to evolving technologies and changing societal values. As we work to develop these frameworks, we must also consider how to implement and enforce them effectively, ensuring that ethical considerations are not just an afterthought but an integral part of AI development and deployment.
Conclusion: Addressing AI Ethics Challenges
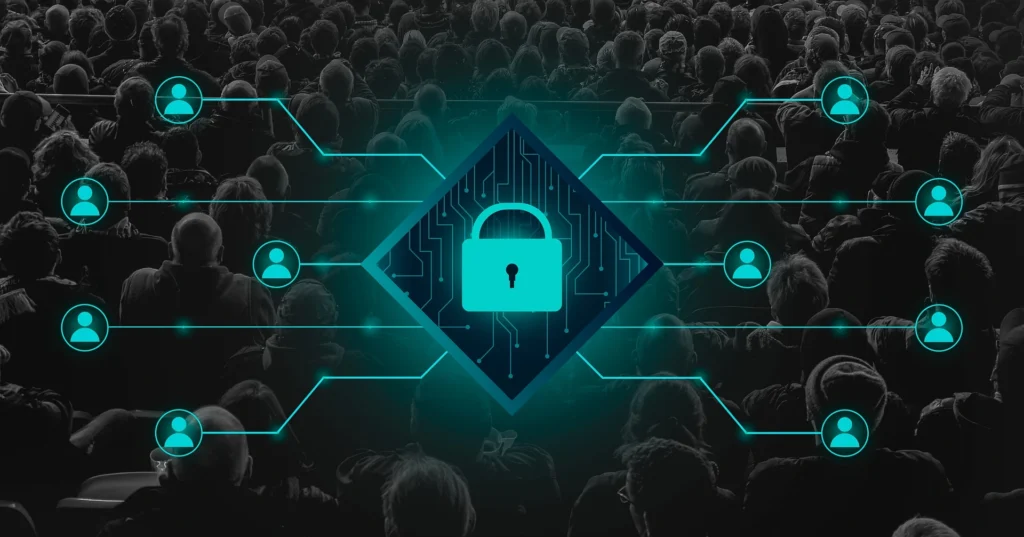
As we navigate the complex landscape of AI ethics challenges, it’s clear that the path forward requires a multifaceted approach. The issues we’ve explored – from bias and fairness to privacy, security, and ethical decision-making – are not just technical problems, but societal ones that demand our collective attention and action. Addressing these AI ethics challenges will require ongoing collaboration between diverse stakeholders, including technologists, ethicists, policymakers, and representatives from various communities.
Education and public awareness play a vital role in tackling AI ethics challenges. As AI becomes increasingly integrated into our daily lives, it’s essential that the general public understands both the potential benefits and risks of these technologies. This knowledge empowers individuals to make informed decisions about their interactions with AI systems and to participate in important societal discussions about the ethical use of AI.
Looking ahead, the field of AI ethics will continue to evolve as new technologies emerge and our understanding of their implications deepens. We must remain vigilant and proactive, continuously reassessing our ethical frameworks and practices. By doing so, we can work towards a future where AI not only enhances our capabilities but also upholds our values and contributes to the greater good of society. While the AI ethics challenges we face are significant, with careful consideration, collaborative effort, and a commitment to ethical principles, we can harness the power of AI responsibly and ethically.